Defended on Decembre 20th 2023
Architectures de Transformer légères pour la reconnaissance de textes manuscrits anciens
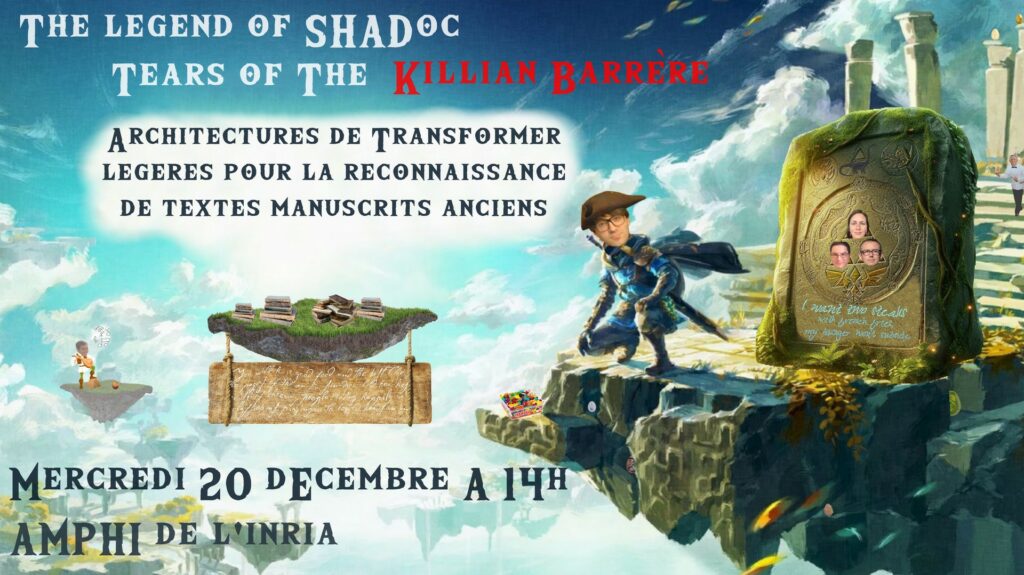
Transformer architectures deliver low error rates but are challenging to train due to limited annotated data in handwritten text recognition. We propose lightweight Transformer architectures to adapt to the limited amounts of annotated handwritten text available. We introduce a fast Transformer architecture with an encoder, processing up to 60 pages per second. We also present architectures using a Transformer decoder to incorporate language modeling into character recognition. To effectively train our architectures, we offer algorithms for generating synthetic data adapted to the visual style of modern and historical documents. Finally, we propose strategies for learning with limited data and reducing prediction errors. Our architectures, combined with synthetic data and these strategies, achieve competitive error rates on lines of text from modern documents. For historical documents, they train effectively with minimal annotated data, surpassing state-of-the-art approaches. Remarkably, just 500 annotated lines are sufficient for character error rates close to 5%.
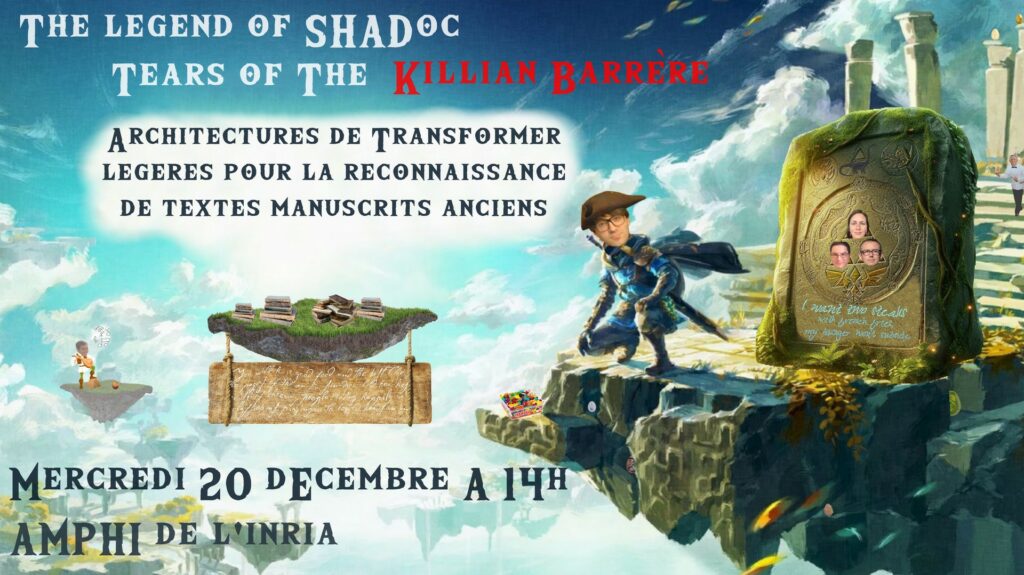
Composition du jury
- Rapporteur – Christophe GARCIA, INSA de Lyon
- Rapporteur – Thierry PAQUET, Université de Rouen Normandie
- Examinateur : Joseph LLADOS, Université polytechnique de Catalogne (Barcelone)
- Examinateur : Harold MOUCHERE, Nantes université
- Directeur de thèse : Bertrand COUASNON, INSA de Rennes
- Co-Directeur de thèse : Aurélie LEMAITRE, université Rennes 2
- Co-encadrant de thèse (invité) : Yann SOULLARD, université Rennes 2